Rosa Galvรฃo – Polytechnic Institute of Setรบbal, School of Business and Administration, Setรบbal, Portugal
Rui Dias – ISG – Business & Economics School, CIGEST, Lisbon, Portugal; ESCAD โ Polytechnic Institute of Lusophony, Lisbon, Portugal
Cristina Palma – Polytechnic Institute of Setรบbal, School of Business and Administration, Setรบbal, Portugal
Paulo Alexandre – Polytechnic Institute of Setรบbal, School of Business and Administration, Setรบbal, Portugal
Sidalina Gonรงalves – Polytechnic Institute of Setรบbal, School of Business and Administration, Setรบbal, Portugal
Keywords:
Artificial intelligence;
Fintech;
Portfolio rebalancing
Abstract: The main purpose of this study is to understand the movements between Fintech and AI stock indices, namely the Global Fintech, Artificial Intelligence & BigData Index (IAIQ), Blockchain Index (ILEGR), Disruptive Technology Index (IDTEC), in order to understand whether they behave as diversifying assets for Indiaโs main stock index (Nifty 50). The results show no evidence that the Fintech and Artificial Intelligence (AI) stock indices, as well as other related indices such as Blockchain and Disruptive Technology, behave as diversifying assets concerning Indiaโs main stock index, the Nifty 50, during the period from January 2020 to January 2024. In conclusion, inยญvestors should adopt a prudent approach when considering including these assets in their portfolios and seek effective diversification through a variety of assets and strategies.
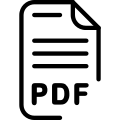
Download full paper
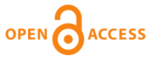
8th International Scientific Conference – EMAN 2024 – Economics and Management: How to Cope With Disrupted Times, Rome, Italy, March 21, 2024, SELECTED PAPERS, published by: Association of Economists and Managers of the Balkans, Belgrade, Serbia; ISBN 978-86-80194-84-4, ISSN 2683-4510, DOI: https://doi.org/10.31410/EMAN.S.P.2024
Creative Commons Nonย Commercial CC BY-NC: This article is distributed under the terms of the Creative Commons Attribution-Non-Commercial 4.0 License (https://creativecommons.org/licenses/by-nc/4.0/) which permits non-commercial use, reproduction and distribution of the work without further permission.ย
REFERENCES
Alaassar, A., Mention, A.-L., & Aas, T. H. (2023). Facilitating innovation in FinTech: a review and research agenda. Review of Managerial Science, 17(1), 33-66. https://doi.org/10.1007/s11846-022-00531-x
Alshater, M. M., Polat, O., El Khoury, R., & Yoon, S.-M. (2024). Dynamic connectedness among regional FinTech indices in times of turbulences. Applied Economics Letters, 31(7), 670-675. https://doi.org/10.1080/13504851.2022.2141443
Bhatnagar, M., รzen, E., Taneja, S., Grima, S., & Rupeika-Apoga, R. (2022). The Dynamic Conยญnectedness between Risk and Return in the Fintech Market of India: Evidence Using the GARCH-M Approach. Risks, 10(11). https://doi.org/10.3390/risks10110209
Breitung, J. (2000). The local power of some unit root tests for panel data. Advances in Econometยญrics. https://doi.org/10.1016/S0731-9053(00)15006-6
Dias, R., Galvรฃo, R., & Alexandre, P. (2024). Precious metals as hedging assets: Evidence from MENA countries. Investment Management and Financial Innovations, 21(1), 157โ167. https://doi.org/10.21511/imfi.21(1).2024.13
Dias, R. M., Chambino, M., Teixeira, N., Alexandre, P., & Heliodoro, P. (2023). Balancing Portfoยญlios with Metals: A Safe Haven for Green Energy Investors ? Energies, 16(20), 7197. https://doi.org/10.3390/en16207197
Dias, R. M. T. S. T., Chambino, M., Alexandre, P., da Palma, C. M., & Almeida, L. (2023). Unveilยญing Bitcoinโs Safe Haven and Hedging Properties Beyond Diversification. In L. Carvalho, C. Silveira, L. Reis, & N. Russo (Eds.), Internet of Behaviors Implementation in Organizaยญtional Contexts (pp. 380-410). IGI Global. https://doi.org/10.4018/978-1-6684-9039-6.ch018
Dias, R. T., Chambino, M., Palma, C., Almeida, L., & Alexandre, P. (2023). Overreaction, underยญreaction, and short-term efficient reaction evidence for cryptocurrencies. In L. Carvalho, C. Silveira, L. Reis, & N. Russo (Eds.), Internet of Behaviors Implementation in Organizationยญal Contexts (pp. 288-312). IGI Global. https://doi.org/10.4018/978-1-6684-9039-6.ch014
Dickey, D., & Fuller, W. (1981). Likelihood ratio statistics for autoregressive time series with a unit root. Econometrica, 49(4), 1057โ1072. https://doi.org/10.2307/1912517
Galvรฃo, R., & Dias, R. (2024). Asymmetric Efficiency: Contrasting Sustainable Energy Indices with Dirty. Financial Economics Letters, 3(1), 22. https://doi.org/10.58567/fel03010002
Ha, L. T. (2023). Dynamic connectedness between FinTech innovation and energy volatility durยญing the war in time of pandemic. Environmental Science and Pollution Research. https://doi.org/10.1007/s11356-023-28089-5
Im, K. S., Pesaran, M. H., & Shin, Y. (2003). Testing for unit roots in heterogeneous panels. Jourยญnal of Econometrics. https://doi.org/10.1016/S0304-4076(03)00092-7
Jarque, C. M., & Bera, A. K. (1980). Efficient tests for normality, homoscedasticity and seriยญal independence of regression residuals. Economics Letters, 6(3), 255โ259. https://doi.org/10.1016/0165-1765(80)90024-5
Le, L. T. N., Yarovaya, L., Ali, M., & Nasir, M. A. (2021). Research in International Business and Finance Did COVID-19 change spillover patterns between Fintech and other asset classes ? Research in International Business and Finance, 58 (August 2020).
Levin, A., Lin, C.-F., & James Chu, C.-S. (2002). Unit root tests in panel data: asymptotic and finite-sample properties. Journal of Econometrics, 108(1), 1-24. https://doi.org/10.1016/s0304-4076(01)00098-7
Li, C., He, S., Tian, Y., Sun, S., & Ning, L. (2022). Does the bankโs FinTech innovation reduce its risk-taking? Evidence from Chinaโs banking industry. Journal of Innovation and Knowlยญedge, 7(3). https://doi.org/10.1016/j.jik.2022.100219
Mumthas, S. (2022). Emerging Trends in Indian FinTech Market. ComFin Research, 10(4). https://doi.org/10.34293/commerce.v10i4.5118ย
Phillips, P. C. B., & Perron, P. (1988). Testing for a unit root in time series regression. Biometrika, 75(2), 335โ346. https://doi.org/10.1093/biomet/75.2.335ย
Santana, T. P., Horta, N. R., Ramos, M. C., Dias, R. M. T. S., Vasconcelos, R. N., da Silva Filho, A. M., & Zebende, G. F. (2023). Interdependence and contagion effects in agricultural comยญmodities markets: A bibliometric analysis, implications, and insights for sustainable develยญopment. In Equilibrium. Quarterly Journal of Economics and Economic Policy (Vol. 18, Isยญsue 4). https://doi.org/10.24136/eq.2023.029ย
Sharma, S., Aggarwal, V., Dixit, N., & Yadav, M. P. (2023). Time and Frequency Connectedness Among Emerging Markets and QGREEN, FinTech and Artificial Intelligence-Based Index: Lessons from the Outbreak of COVID-19. Vision. https://doi.org/10.1177/09722629221141553ย
Siska, E. (2022). Financial Technology (FinTech) and Its Impact on Financial Performance of Islamic Banking. ARBITRASE: Journal of Economics and Accounting, 2(3). https://doi.org/10.47065/arbitrase.v2i3.338ย
Teixeira, N., Dias, R. T., Pardal, P., & Horta, N. R. (2023). Financial Integration and Comoveยญments Between Capital Markets and Oil Markets: An Approach During the Russian Invaยญsion of Ukraine in 2022. In I. Lisboa, N. Teixeira, L. Segura, T. Krulickรฝ, & V. Machovรก (Eds.), Handbook of Research on Acceleration Programs for SMEs (pp. 240-261). IGI Globยญal. https://doi.org/10.4018/978-1-6684-5666-8.ch013ย
Wang, L., Guan, L., Ding, Q., & Zhang, H. (2023). Asymmetric impact of COVID-19 news on the connectedness of the green energy, dirty energy, and non-ferrous metal markets. Energy Economics, 126. https://doi.org/10.1016/j.eneco.2023.106925ย
Yadav, V. K. (2023). Analysing Opportunities and Obstacles of FINTECH in Indian Financial Market. International Journal of Research in Education Humanities and Commerce, 04(01). https://doi.org/10.37602/ijrehc.2023.4103ย
Zhou, H., & Li, S. (2022). Effect of COVID-19 on risk spillover between Fintech and traditional fiยญnancial industries. Frontiers in Public Health, 10. https://doi.org/10.3389/fpubh.2022.97980ย
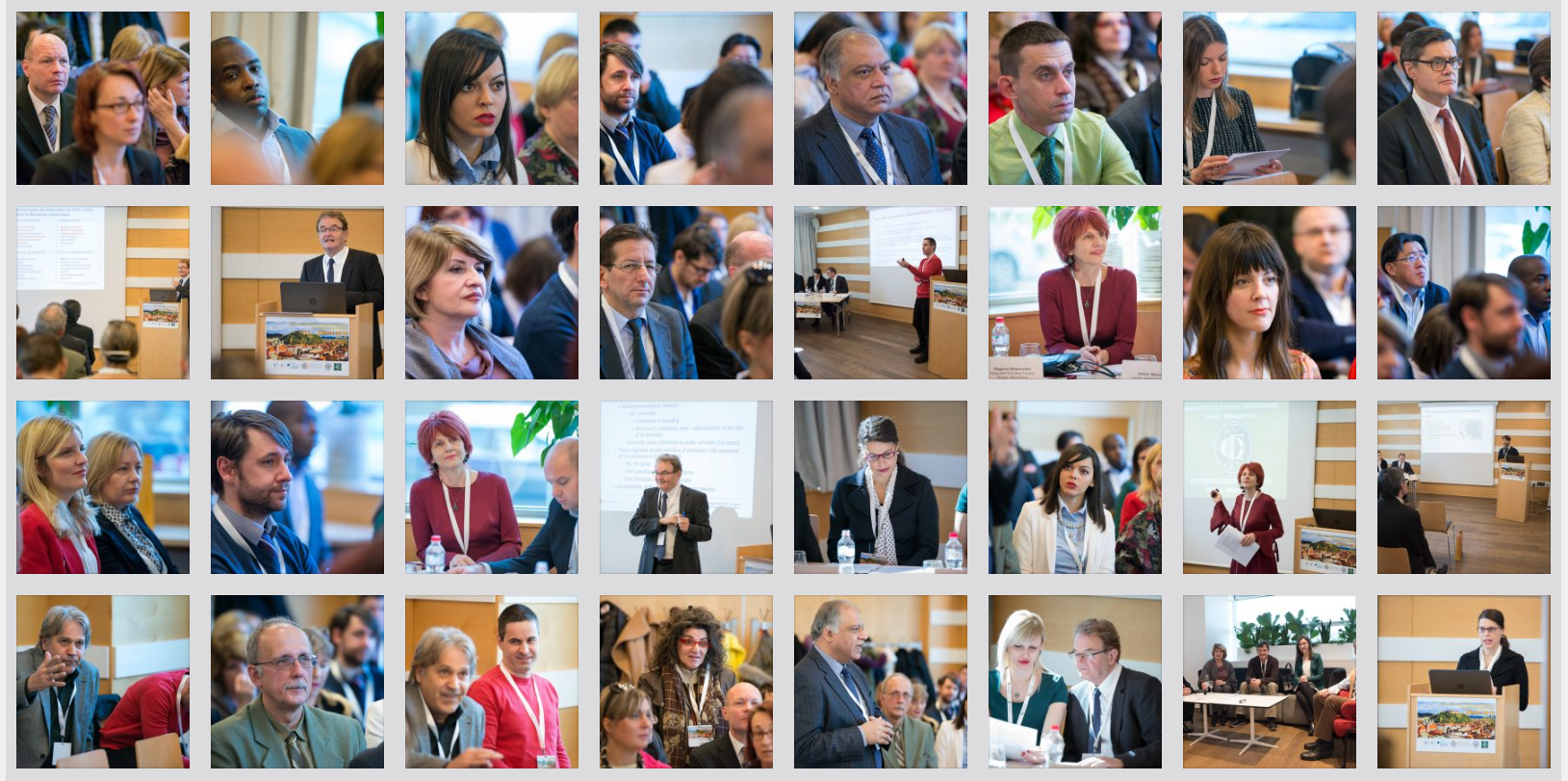