Nicole Horta – ESCE, Instituto Politรฉcnico de Setรบbal, Portugal
Mariana Chambino – ESCE, Instituto Politรฉcnico de Setรบbal, Portugal
Rui Dias – ESCE, Instituto Politรฉcnico de Setรบbal, Portugal; Center for Studies and Advanced Training in Management and Economics (CEFAGE), University of รvora, Portugal
Keywords:
Clean energy;
2020 and 2022 events;
Co-movements;
Diversification of portfolios
Abstract: The study of the changes between the Energy Fuels Index, S&P Global Clean Energy Index, iShares Global Clean Energy ETF, iShares Global Energy (SWX) ETF, as well as the changes in the prices of crude oil (BRENT), gold (DJ), and natural gas (DG) was deemed extremely relevant given the imยญportance and emergence of clean energies in the global landscape, as well as the need to develop more empirical studies, especially confirmative studยญies on the financial dynamics in these markets. The daily returns under analยญysis exhibit negative and leptokurtic asymmetry rather than a normal distriยญbution. Comparatively, the pre-crisis linkages between the markets for dirty and clean energy are in favor of global portfolio diversification since those low levels of dependence are appropriate to reduce investor exposure to risk. The crude oil market already exhibited a significant effect on the clean enerยญgy markets during the Stress subperiod, particularly on the Clean Energy Fuels Index, the iShares Global Clean Energy ETF, and the iShares Global Clean Enยญergy (SWX) ETF. It should be highlighted that the clean energy markets have also increased their impact on the markets for gold and dirty energy (crude oil and natural gas). The findings point to an increase in comovements between the examined indices and the events of 2020 and 2022. These results decrease the possibility that clean energy markets will serve as a portfolio diversificaยญtion substitute for the gold and dirty energy markets. For investors and finanยญcial analysts who are interested in understanding how the various sectors of the energy market interact, these results may also have consequences. These results can enable a more precise forecast of energy market trends and more informed investment decisions by offering a more detailed knowledge of the link between clean and dirty energy prices.
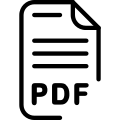
Download full paper
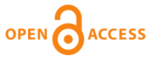
7th International Scientific Conference – EMAN 2023 – Economics and Management: How to Cope With Disrupted Times, Ljubljana, Slovenia, March 23, 2023, CONFERENCE PROCEEDINGS, published by: Association of Economists and Managers of the Balkans, Belgrade, Serbia; ISBN 978-86-80194-69-1, ISSN 2683-4510, DOI: https://doi.org/10.31410/EMAN.2023
Creative Commons Nonย Commercial CC BY-NC: This article is distributed under the terms of the Creative Commons Attribution-Non-Commercial 4.0 License (https://creativecommons.org/licenses/by-nc/4.0/) which permits non-commercial use, reproduction and distribution of the work without further permission.ย
Angelini, E., Birindelli, G., Chiappini, H., & Foglia, M. (2022). Clean energy indexes and brown assets: an analysis of tail risk spillovers through the VAR for VaR model. Journal of Susยญtainable Finance and Investment. https://doi.org/10.1080/20430795.2022.2105788ย
Basoglu, M. S., Korkmaz, T., & Cevik, E. I. (2014). London metal exchange: Causality relationยญship between the price series of non-ferrous metal contracts. International Journal of Ecoยญnomics and Financial Issues, 4(4).
Breitung, J. (2000). The local power of some unit root tests for panel data. Advances in Econoยญmetrics. https://doi.org/10.1016/S0731-9053(00)15006-6
Chen, J., Wang, Y., & Ren, X. (2022). Asymmetric effects of non-ferrous metal price shocks on clean energy stocks: Evidence from a quantile-on-quantile method. Resources Policy, 78. https://doi.org/10.1016/j.resourpol.2022.102796ย
Dias, R., Pereira, J. M., & Carvalho, L. C. (2022). Are African Stock Markets Efficient? A Comparaยญtive Analysis Between Six African Markets, the UK, Japan and the USA in the Period of the Panยญdemic. Naลกe Gospodarstvo/Our Economy, 68(1), 35โ51. https://doi.org/10.2478/ngoe-2022-0004ย
Dias, R., & Santos, H. (2020). the Impact of Covid-19 on Exchange Rate Volatility: an Econoยญphysics Approach. 6th LIMEN Conference Proceedings (Part of LIMEN Conference Colยญlection), 6(July), 39โ49. https://doi.org/10.31410/limen.2020.39ย
Dias, R., Teixeira, N., Machova, V., Pardal, P., Horak, J., & Vochozka, M. (2020). Random walks and market efficiency tests: Evidence on US, Chinese and European capital marยญkets within the context of the global Covid-19 pandemic. Oeconomia Copernicana, 11(4). https://doi.org/10.24136/OC.2020.024ย
Dias, R. T., & Carvalho, L. (2021a). Foreign Exchange Market Shocks in the Context of the Global Pandemic (COVID-19). 359โ373. https://doi.org/10.4018/978-1-7998-6643-5.ch020ย
Dias, R. T., & Carvalho, L. (2021b). The Relationship Between Gold and Stock Markets During the COVID-19 Pandemic. May, 462โ475. https://doi.org/10.4018/978-1-7998-6643-5.ch026ย
Dias, R. T., Pardal, P., Teixeira, N., & Horta, N. R. (2022). Tail Risk and Return Predictabilยญity for Europeโs Capital Markets: An Approach in Periods of the 2020 and 2022 Crises. Advances in Human Resources Management and Organizational Development, 281-298. https://doi.org/10.4018/978-1-6684-5666-8.ch015ย
Dickey, D., & Fuller, W. (1981). Likelihood ratio statistics for autoregressive time series with a unit root. Econometrica, 49(4), 1057โ1072. https://doi.org/10.2307/1912517ย
Foglia, M., Angelini, E., & Huynh, T. L. D. (2022). Tail risk connectedness in clean enerยญgy and oil financial market. Annals of Operations Research. https://doi.org/10.1007/s10479-022-04745-wย
Granger, C. W. J., & Newbold, P. (1974). Spurious regressions in econometrics. Journal of Econometrics, 2(2), 111โ120. https://doi.org/10.1016/0304-4076(74)90034-7
Guedes, E. F., Santos, R. P. C., Figueredo, L. H. R., Da Silva, P. A., Dias, R. M. T. S., & Zebende, G. F. (2022). Efficiency and Long-Range Correlation in G-20 Stock Indexes: A Sliding Windows Approach. Fluctuation and Noise Letters. https://doi.org/10.1142/S021947752250033Xย ย
He, Y. X., Jiao, Z., & Yang, J. (2018). Comprehensive evaluation of global clean energy developยญment index based on the improved entropy method. Ecological Indicators, 88. https://doi.org/10.1016/j.ecolind.2017.12.013ย ย
Herranz, E. (2017). Unit root tests. In Wiley Interdisciplinary Reviews: Computational Statisยญtics. https://doi.org/10.1002/wics.1396ย
Horta, N., Dias, R., Revez, C., & Alexandre, P. (2022). CRYPTOCURRENCIES AND G7 CAPIยญTAL MARKETS INTEGRATE IN PERIODS OF EXTREME VOLATILITY ? 10(3), 121โ130.
Horta, N., Dias, R., Revez, C., Heliodoro, P., & Alexandre, P. (2022). Spillover and Quantitaยญtive Link Between Cryptocurrency Shocks and Stock Returns: New Evidence From G7 Countries. Balkans Journal of Emerging Trends in Social Sciences, 5(1), 1โ14. https://doi.org/10.31410/balkans.jetss.2022.5.1.1-14ย ย
Im, K. S., Pesaran, M. H., & Shin, Y. (2003). Testing for unit roots in heterogeneous panels. Journal of Econometrics. https://doi.org/10.1016/S0304-4076(03)00092-7ย
Jarque, C. M., & Bera, A. K. (1980). Efficient tests for normality, homoscedasticity and seยญrial independence of regression residuals. Economics Letters, 6(3), 255โ259. https://doi.org/10.1016/0165-1765(80)90024-5ย ย
Levin, A., Lin, C.-F., & James Chu, C.-S. (2002). Unit root tests in panel data: asymptotic and finite-sample properties. Journal of Econometrics, 108(1), 1-24. https://doi.org/10.1016/s0304-4076(01)00098-7ย ย
Liu, N., Liu, C., Da, B., Zhang, T., & Guan, F. (2021). Dependence and risk spillovers between green bonds and clean energy markets. Journal of Cleaner Production, 279. https://doi.org/10.1016/j.jclepro.2020.123595ย
Mighri, Z., Ragoubi, H., Sarwar, S., & Wang, Y. (2022). Quantile Granger causality between US stock market indexes and precious metal prices. Resources Policy, 76. https://doi.org/10.1016/j.resourpol.2022.102595ย ย
Pandey, D. K., Kumar, R., & Kumari, V. (2023). Glasgow climate pact and the global clean energy index constituent stocks. International Journal of Emerging Markets. https://doi.org/10.1108/IJOEM-05-2022-0815ย ย
Pardal, P., Dias, R., Teixeira, N., & Horta, N. (2022). The Effects of Russiaโ s 2022 Invasion of Ukraine on Global Markets : An Analysis of Particular Capital and Foreign Exchange Markets. https://doi.org/10.4018/978-1-6684-5666-8.ch014ย
Pardal, P., Dias, R. T., Santos, H., & Vasco, C. (2021). Central European Banking Sector Inteยญgration and Shocks During the Global Pandemic (COVID-19). June, 272โ288. https://doi.org/10.4018/978-1-7998-6926-9.ch015ย
Perron, P., & Phillips, P. C. B. (1988). Testing for a Unit Root in a Time Series Regression. Biยญometrika, 2(75), 335โ346. https://doi.org/10.1080/07350015.1992.10509923ย
Reboredo, J. C., & Ugolini, A. (2018). The impact of energy prices on clean energy stock pricยญes. A multivariate quantile dependence approach. Energy Economics, 76. https://doi.org/10.1016/j.eneco.2018.10.012ย ย
Shafiullah, M., Chaudhry, S. M., Shahbaz, M., & Reboredo, J. C. (2021). Quantile causality and dependence between crude oil and precious metal prices. International Journal of Finance and Economics, 26(4). https://doi.org/10.1002/ijfe.2119ย
Tahai, A., Rutledge, R. W., & Karim, K. E. (2004). An examination of financial integration for the group of seven (G7) industrialized countries using an I (2) cointegration model. Apยญplied Financial Economics, 14(5), 327โ335. https://doi.org/10.1080/0960310042000211597ย
Teixeira, N., Dias, R., & Pardal, P. (2022). The gold market as a safe haven when stock markets exhibit pronounced levels of risk : evidence during the China crisis and the COVID-19 pandemic. April, 27โ42.
Teixeira, N., Dias, R. T., Pardal, P., & Horta, N. R. (2022). Financial Integration and Comoveยญments Between Capital Markets and Oil Markets: An Approach During the Russian Invaยญsion of Ukraine in 2022. Advances in Human Resources Management and Organizational Development, 240-261. https://doi.org/10.4018/978-1-6684-5666-8.ch013ย
Tiwari, A. K., Abakah, E. J. A., Yaya, O. O. S., & Appiah, K. O. (2023). Tail risk dependence, co-movement and predictability between green bond and green stocks. Applied Economยญics, 55(2). https://doi.org/10.1080/00036846.2022.2085869ย
Yahya, M., Ghosh, S., Kanjilal, K., Dutta, A., & Uddin, G. S. (2020). Evaluation of cross-quanยญtile dependence and causality between non-ferrous metals and clean energy indexes. Enยญergy, 202. https://doi.org/10.1016/j.energy.2020.117777ย
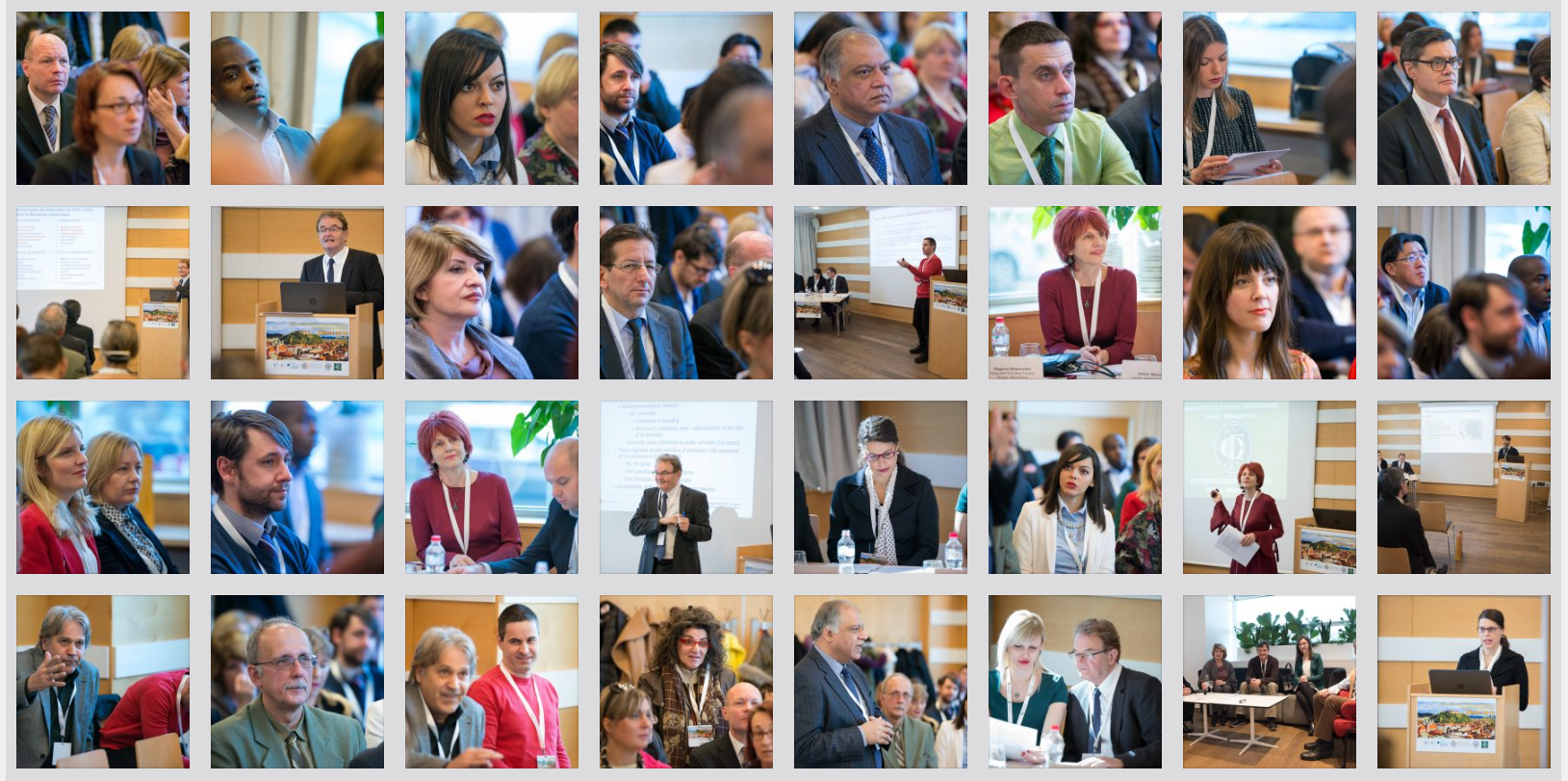